SUCCESS CASE:
Terminal 6
Machine Learning to automate rail monitoring
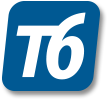
About Terminal 6
Located on the banks of the Paraná River, Terminal 6 (T6) is the most important agro-industrial export complex in Latin America. The port receives more than 80,000 tons of raw materials daily, which are stored, transformed and finally dispatched to various parts of the world.
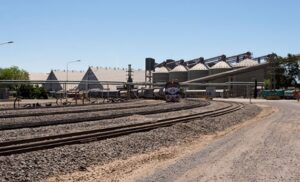
The Challenge: Non-standard wagon codes
Transport by train represents 33% of the total. The terminal receives between 8 and 10 trains loaded with grain daily, counting approximately 50 carriages labelled with their respective identification codes. The wagons must be identified at least two times, when they enter the terminal and when they pass through the weighing scale to be then imputed into the information management systems. This process, done manually, had to be digitalized, given the data collection process represented a bottleneck for T6 and suffered the risk errors.
The wagon codes found on these trains are not ISO standards, quite the opposite, unique to this use case, and therefore no robust “from the shelf” solution was available. The system should be able to recognize two different types of codes, in addition to facing quite challenging scenarios in terms of the visibility/readability of these codes, which are often dirty or damaged significantly.
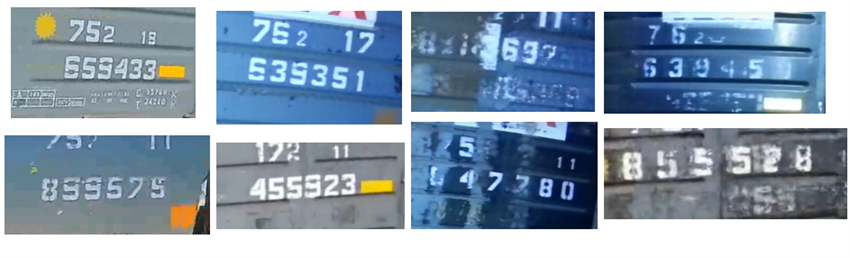
The Solution: Machine Learning Technology
The proposed solution from AllRead involves the deployment of two different AI models. The first is the model devoted to detecting and recognizing the wagon codes (A), while the second model is used to detect wagon separators (couplings) (B). Even though some wagon codes are illegible, we can assemble the correct train map by the combination of those two models in parallel.
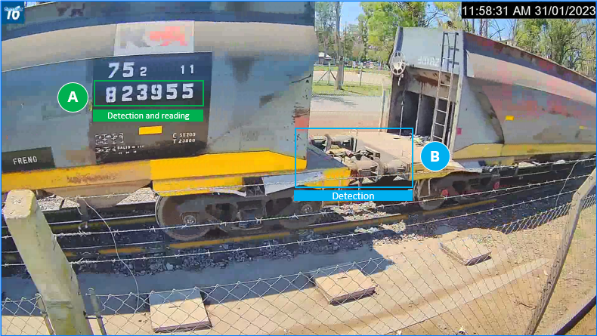
In order to quickly provide a working solution to our client, we first deployed our generic scene text recognition models, able to spot and recognize whichever text there is present in the images. An ad-hoc post-processing step then identified the wagon codes from the rest of the extracted text.
This initial implementation yielded results of around 80%. Some of the codes were not correctly detected, and the font used to print the codes in the wagons provoked that often digits ‘5’, ‘6’ and ‘9’ were mistakenly recognized (see image above). Although few additional data was available, the models needed to be retrained to tackle the data and model drift that we were facing. We put in place two iterations of the MLOps loop, that involved gathering, but mostly synthetizing new training data, fine-tuning and domain adaptation of our Deep Learning models for text detection and text recognition and performance monitoring. We finally deployed a tailor-made solution for those specific wagon codes that provided accuracies beyond 96% recognition.
Minimum hardware requirements and ease of installation
The solution proposed by AllRead was perfectly suited what T6 was looking for. After some iterations over the initial deployed models, and regardless of the challenging scenario, thanks to the robustness of the software and its AI models, AllRead stays true to its philosophy: always ensure minimum hardware requirements and ease of installation: two cameras and two light spots fixed on posts on both side of the lane, and connected to one on premise server.
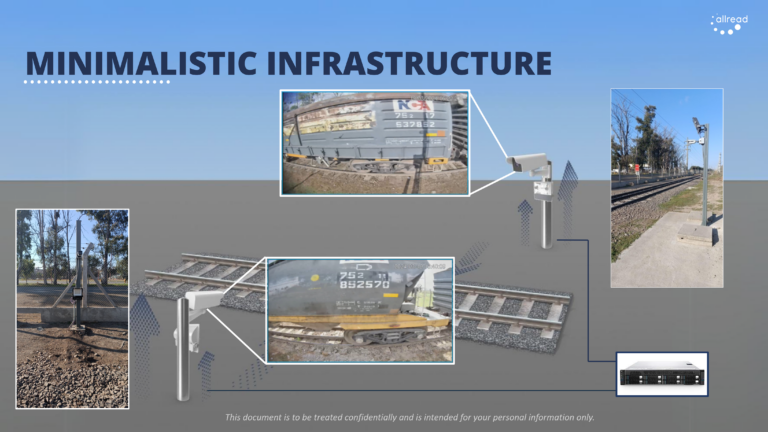
With the support of Generática, the port’s technology consultants and Partner of AllRead, the solution has been integrated into the information systems and daily tasks of the operators. Considering that at the beginning of 2024, while the weighing process was digital, the monitoring of trains was done manually (that is, for each train that entered, the notes were taken manually to be linked to their respective weights afterwards), one can assume the significant gain in efficiency of automating the data collection and consolidation.
The Results: Customised OCR solution
In addition, through the dashboard provided by AllRead, control and, when required, correction of the data is enabled, with no need for the operator to move from its workplace. Interestingly, the impact of the automation is also appreciated on various other processes of T6, like the verification of the reported weight, invoicing, etc., freeing time of the operators to concentrate on tasks with greater added value.
In the light of the benefits of this project, AllRead and T6 already glimpse the scalability of this solution, both the same terminal and in other Terminals of the group. Considering the AI models have been customized and the system prepared for integration with the current TOS of T6 with Generatica, rolling out the solution on various sites of the Terminal Operator requires minimal additional efforts.
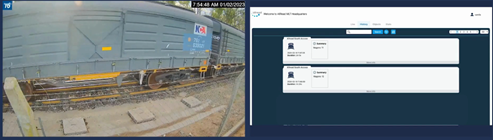
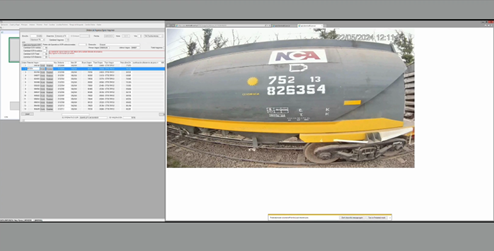
If you would like to know more about this success story, contact our team.